Computer networks are a critical component of modern and advanced digital applications present all around the world. However, anytime the linkages become numerous enough to support the growing organization and the management of the networks becomes a real big issue. So this is where Machine Learning (ML) in computer networks comes into play. Implementing ‘learning’ algorithms ML makes computer networks wiser, swifter, and safer with every passing moment.
So in this blog series, we will look at how and where Deep Learning or Machine Learning is making its presence felt in computer networks.
What is Machine Learning in Computer Networks?
Still, let me explain what ML is before the run of applications and examples. Machine Learning is a subset of Artificial intelligence in which a system is allowed to learn from data without being programmed to do so. Moreover, it uses algorithms to:
- Analyze patterns in data
- Make predictions
- Improve decisions over time
In the computer networks area, researchers use ML to handle large amounts of data, making the network smart and active.
Traffic Analysis and Management
Current computer networks deal with large numbers of data within the midst of a second. As its efficient management cannot be overstressed, this is because traffic is the key reason for congestion threats. So ML plays a key role in:
1. Detecting Traffic Patterns
Traffic flow is analyzed by ML for a powerful selection of normal traffic habits and anomalous traffic activity. For example, it can distinguish between users who traffic during highly busy hours and those who traffic during other non-busy periods.
2. Predicting Network Bottlenecks
The main goal of using the methodology is to be able to identify network bottlenecks that have a high probability of occurring. So in the case of predictive models, ML can anticipate when the traffic density might be high. So that networks can be scaled up.
3. Critical Traffic Preservation
ML can sort and allocate data meaning high-priority cybersecurity services such as video calls or funds transfers get a better medium than low-priority ones such as software updates.
For example, traffic is self-regulated by the materialization of the data stream and traffic flow. So the use of this materialization is achieved through ML.
Enhancing Network Security
Security is an area of concern that networks used in computers pose. Phishing scams, malware, and Distributed Denial of Service (DDoS) are some of the common forms of invasions that are getting complex. So ML helps in:
1. Identifying Threats
It identifies that malicious activities of the past are used to train an ML model. For instance, they can recognize such signs as account sign-in or data retrieving by unknown users.
2. Responding to Incidents
ML algorithms are capable of everything once a threat has been identified. So those systems can react on their own they might shut down aggressive IP addresses or quarantine devices on the network.
3. Predicting Future Attacks
Unlike other systems, ML analyzes similar occurrences in the past and calculates the risks essential for offering precautions.
For instance, banks apply ML to check the authenticity of most transactions in the market to protect customers’ money.
Optimizing Network Performance
Hence, networks have to perform at high capacity to satisfy the user’s expectation. So ML improves performance through automation tasks and ensuring efficient use of resources:
1. Dynamic Resource Allocation
Unlike other service providers who permanently assign resources such as bandwidth and server space, ML dynamically assigns them according to usage.
2. Load Balancing
It balances the traffic that runs on the network so that the servers would not get loaded thus increasing reliability.
3. Predictive Maintenance
H/W or SW: ML analyzes them and points out the time when they are going to fail, which makes repair possible. Moreover, Google and Amazon widely apply ML in their data centers to guarantee the highest availability and productivity.
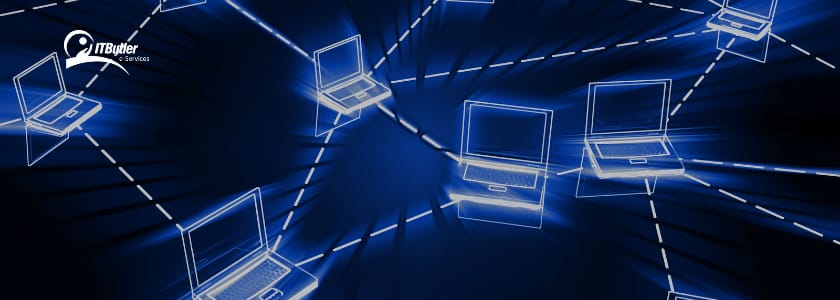
IoT and Edge Computing
In the current world, information has connected billions, and probably millions, of things to networks. So managing these devices and their data is a complex task, but ML simplifies it:
1. Monitoring Devices
An ML primarily looks at optimizing the IoT devices’ functionality since it focuses on their performance.
2. Data Processing at the Edge
Whereas many other techniques send all the information to a few superordinate servers. ML handles data locally within the network border, thereby minimizing latency and bandwidth consumption.
3. IoT-based Predictive Maintenance
ML predicts exactly when an IoT device will develop a fault, allowing technicians to repair it before it causes a problem. Alexa and Google Nest as smart home systems operate devices with the help of ML.
Self-Healing Networks
An ideal self-healing network can self-diagnose, and self-heal without human intervention and provides high availability. So ML enables this by:
1. Detecting Problems
These ML algorithms are also always on the lookout for problems such as slow connection or wrong devices.
2. Automated Troubleshooting
When a problem is detected, ML applies solutions which, may include changing traffic patterns or rebooting problematic devices.
3. Continuous Improvement
However, the system has the capability of adopting lessons from past incidents in solving similar problems in the future.
Lastly, self-healing networks are more important in large organizations. Because decentralized identification and correction of network problems would be impractical.
Energy Efficiency
Power usage becomes a core issue of concern to the networks particularly in data centers. As ML helps reduce energy usage by:
1. Optimizing Power Usage
ML enables a device or server to function only when required, thus reducing power consumption.
2. Efficient Cooling
ML regulates data center temperatures to deliver an optimum environment to the server without wasting energy.
3. Predicting Energy Needs
ML predicts energy needs by tracking the activities on the network thereby enabling better planning.
For instance, Google’s power air conditioning infrastructure in its data centers employs the use of ML to cut power expenditure to as little as 40%.
Conclusion
ML in computer networks is making it intelligent, optimized, and secure using state-of-the-art. As applications range from traffic control and security to energy usage, and even the possibility of responding intelligently to faults. These are virtually limitless possibilities. With the increased development of technology, we will observe that ML is going to revolutionize the future of networking. We have not yet experienced some of the challenges that this technique may solve.
Engineers, business owners, or just enthusiasts, need to learn how those ML in computer networks works. That is not just about optimizing networks today, but about creating the platforms for the smart networks of the future.